Introduction
In recent years, market volatility has become a defining feature of the global stock market landscape. Whether driven by geopolitical tensions, economic uncertainty, or unexpected global events such as pandemics, volatility presents both challenges and opportunities for investors. While stock market fluctuations often evoke fear and uncertainty, they also create potential for significant returns—if approached with the right strategies and tools.
One of the most powerful ways to navigate such volatility is through data analytics. By utilizing advanced data analysis techniques, investors can better understand market trends, predict price movements, and make more informed investment decisions. With the vast amount of data available today, ranging from historical prices to social media sentiment, the ability to process and analyze data has never been more crucial in formulating effective investment strategies.
In this article, we will explore how data analytics can be used to enhance decision-making in the stock market, particularly in times of increased volatility. We will discuss various data sources, analytical methods, and how investors can leverage them to optimize their portfolios and improve their chances of success.
1. Understanding Stock Market Volatility: Causes and Implications
Before diving into the specifics of how data analytics can be used, it’s essential to understand what causes stock market volatility and why it poses challenges to investors.
1.1 What is Market Volatility?
Market volatility refers to the degree of variation in the price of a financial instrument (such as a stock or index) over time. High volatility typically means that the price swings up or down sharply within a short period, while low volatility indicates more stable, gradual price movements.
Volatility can be caused by various factors, including:
- Macroeconomic events: Changes in interest rates, inflation, GDP growth, or employment data can lead to market fluctuations.
- Geopolitical tensions: Political instability, trade wars, or international conflicts can create uncertainty, influencing investor sentiment.
- Corporate earnings reports: A company’s financial performance can affect its stock price, particularly when results differ significantly from analysts’ expectations.
- Market sentiment: Investor psychology, including reactions to news and rumors, can drive short-term volatility, particularly in speculative markets.
Volatility is a natural part of the stock market, but it can create challenges for investors who aim to predict short-term price movements or maintain portfolio stability.
2. The Role of Data Analytics in Navigating Market Volatility
In times of heightened market volatility, investors are presented with two key tasks: first, analyzing market trends to identify opportunities, and second, predicting future movements to manage risk. Data analytics can significantly enhance these tasks by offering deeper insights into market dynamics, investor behavior, and asset performance.
2.1 Key Data Sources for Stock Market Analysis
The foundation of effective data analysis lies in identifying and collecting the right data. Some of the primary data sources used by investors for stock market analysis include:
- Historical Price Data: Historical prices of stocks, indices, and other financial instruments are fundamental to technical analysis. This data includes opening/closing prices, high/low prices, and trading volume, and helps investors identify price patterns and trends over time.
- Financial Statements: Companies’ financial reports (such as income statements, balance sheets, and cash flow statements) provide insight into their financial health. These documents are crucial for fundamental analysis, enabling investors to assess profitability, solvency, and operational efficiency.
- Market Indicators: Economic indicators like interest rates, inflation rates, and GDP growth are often used to gauge the broader economic environment. Tools like the VIX (Volatility Index) provide direct insight into market sentiment and fear.
- Sentiment Analysis: Social media platforms, news articles, and analyst reports can be mined to gauge investor sentiment. By analyzing the tone and frequency of discussions around a particular stock, analysts can understand market mood and anticipate short-term price movements.
- Alternative Data: Data from non-traditional sources like satellite imagery (tracking supply chain disruptions), web traffic analytics, or even credit card transaction data can provide unique insights into a company’s operations or broader market trends.
2.2 Analytical Techniques to Enhance Investment Decisions
Once relevant data is collected, various analytical techniques can be employed to make sense of the information. Some of the most commonly used techniques in stock market data analysis include:
- Technical Analysis: This involves analyzing historical price and volume data to identify trends, chart patterns, and key support/resistance levels. Common technical analysis tools include:
- Moving Averages (MA): Smooth out price data to identify trends.
- Relative Strength Index (RSI): Measures whether a stock is overbought or oversold.
- Bollinger Bands: Help assess volatility by measuring the range within which a stock’s price typically fluctuates.
- Fundamental Analysis: Investors use fundamental analysis to assess a company’s intrinsic value based on its financials and economic environment. Key metrics include:
- Earnings per Share (EPS): Indicates a company’s profitability.
- Price-to-Earnings Ratio (P/E): Used to determine if a stock is overvalued or undervalued.
- Return on Equity (ROE): Measures how effectively a company generates profit from its equity.
- Quantitative Analysis: This involves using mathematical models and algorithms to predict stock price movements based on historical data. Quantitative models may also incorporate machine learning (ML) to identify complex patterns that might not be immediately apparent to human analysts.
- Sentiment Analysis and Natural Language Processing (NLP): Sentiment analysis tools use NLP to scan news articles, social media posts, earnings calls, and analyst reports to gauge investor sentiment. A positive sentiment may indicate buying opportunities, while a negative sentiment could signal potential sell-offs.
- Machine Learning and AI: Advanced techniques such as supervised learning, unsupervised learning, and reinforcement learning can be used to model and predict future stock price movements. These algorithms can recognize complex, non-linear relationships in data that traditional methods might miss.
3. Strategies for Leveraging Data Analysis in Volatile Markets
Now that we’ve explored the types of data and analytical techniques available to investors, let’s look at practical strategies to leverage data analytics in volatile markets.
3.1 Identify and Exploit Short-Term Market Inefficiencies
Market volatility often creates short-term mispricings—situations where stocks are temporarily overvalued or undervalued due to panic or exuberance. By using data analytics to identify these inefficiencies, investors can position themselves to capitalize on mean reversion or trends that are likely to correct in the future.
- Example: If sentiment analysis tools reveal that a company’s stock has been unfairly penalized by negative news, investors may decide to buy the stock at a lower price, anticipating a rebound once the market rationalizes the situation.
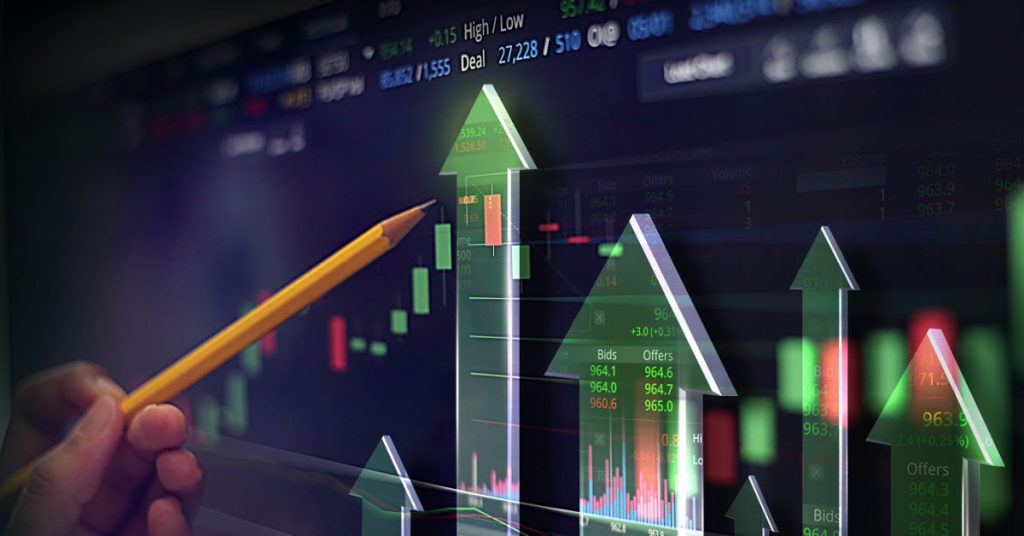
3.2 Implement Risk Management through Predictive Analytics
In times of high volatility, risk management becomes critical. Predictive analytics can be used to anticipate potential downturns or upturns, allowing investors to hedge or adjust their positions proactively.
- Example: Machine learning algorithms can analyze historical price data to identify patterns that precede significant price movements, allowing investors to sell or hedge positions before a drop, or buy in advance of a price increase.
3.3 Use Real-Time Data to Monitor Market Conditions
In volatile markets, things can change quickly. Real-time data, such as live market prices, sentiment indicators, and economic data releases, can help investors stay ahead of shifts in market conditions.
- Example: A sudden shift in sentiment driven by breaking news or earnings reports can be detected and acted upon immediately using sentiment analysis tools and real-time price monitoring.
3.4 Optimize Portfolio with Diversification Models
Data analytics can help investors design more resilient portfolios by optimizing the asset allocation based on various factors such as risk tolerance, expected return, and correlation between assets.
- Example: A portfolio that is heavily weighted toward growth stocks may be more susceptible to sharp declines during a correction. By using risk models and correlation matrices, investors can adjust the portfolio to include a mix of asset types, such as bonds, commodities, and real estate, to mitigate potential losses.
4. Conclusion: Turning Volatility into Opportunity with Data-Driven Decisions
While stock market volatility can be unnerving, it also presents unique opportunities for investors who are equipped with the right tools. By leveraging data analytics, investors can gain a deeper understanding of market trends, make more informed predictions, and enhance their ability to navigate unpredictable environments.
To capitalize on these opportunities, investors need to:
- Gather comprehensive data from diverse sources.
- Apply advanced analytical techniques to interpret this data effectively.
- Use predictive models and real-time information to make timely decisions.
In the age of big data and advanced analytics, the ability to analyze and act on information quickly is no longer just a competitive advantage—it is a necessity. With the right approach, volatility can become less of a risk and more of a valuable opportunity for savvy investors.